In today’s digital age, where data is generated at an unprecedented rate, businesses and industries increasingly rely on real-time data processing for immediate insights and decision-making. It is one of the key technological advancements transforming how data is processed, analyzed, and utilized in real time. By pushing data processing closer to the source, edge computing reduces latency, increases efficiency, and offers solutions to industries ranging from healthcare to manufacturing.
In this article, we will explore the evolution of edge computing, its benefits, use cases, and how it is reshaping real-time data processing.
Introduction to Edge Computing
The rapid expansion of Internet of Things (IoT) devices, smart sensors, and data-driven applications has led to a surge in the demand for faster data processing. Centralized data processing—often reliant on cloud computing—has its limitations, particularly when it comes to real-time applications. It provides a powerful alternative by decentralizing data processing and bringing it closer to the devices that generate the data, ensuring near-instantaneous processing and reduced latency.
The Evolution of Data Processing: From Centralized to Distributed
Traditionally, most data processing was performed on centralized servers in data centers, often far from the devices or sensors collecting the data. As the amount of data increased real-time processing became critical in industries. Such as healthcare, manufacturing, and transportation, it became clear that centralized systems couldn’t keep up. This challenge gave rise to edge computing. Where data is processed closer to the “edge” of the network, minimizing delays and improving the overall efficiency of data handling.
What is Edge Computing?
It refers to the practice of processing data at or near the location where it is generated, rather than relying on a centralized data center. By processing data locally on edge devices—such as sensors, IoT devices, or routers—the need for constant back-and-forth communication with the cloud is minimized. This enables real-time data analysis and decision-making with minimal latency.
Why Real-Time Data Processing Matters
In many industries, real-time data processing is crucial. For instance, autonomous vehicles need to make split-second decisions based on sensor data to ensure safety. Similarly, in industrial settings, machines may need to adjust their operations instantly based on feedback from sensors. Edge computing enables these applications by providing near-instant data analysis and response, avoiding delays caused by sending data to and from a distant data center.
Key Benefits of Edge Computing for Real-time Data Processing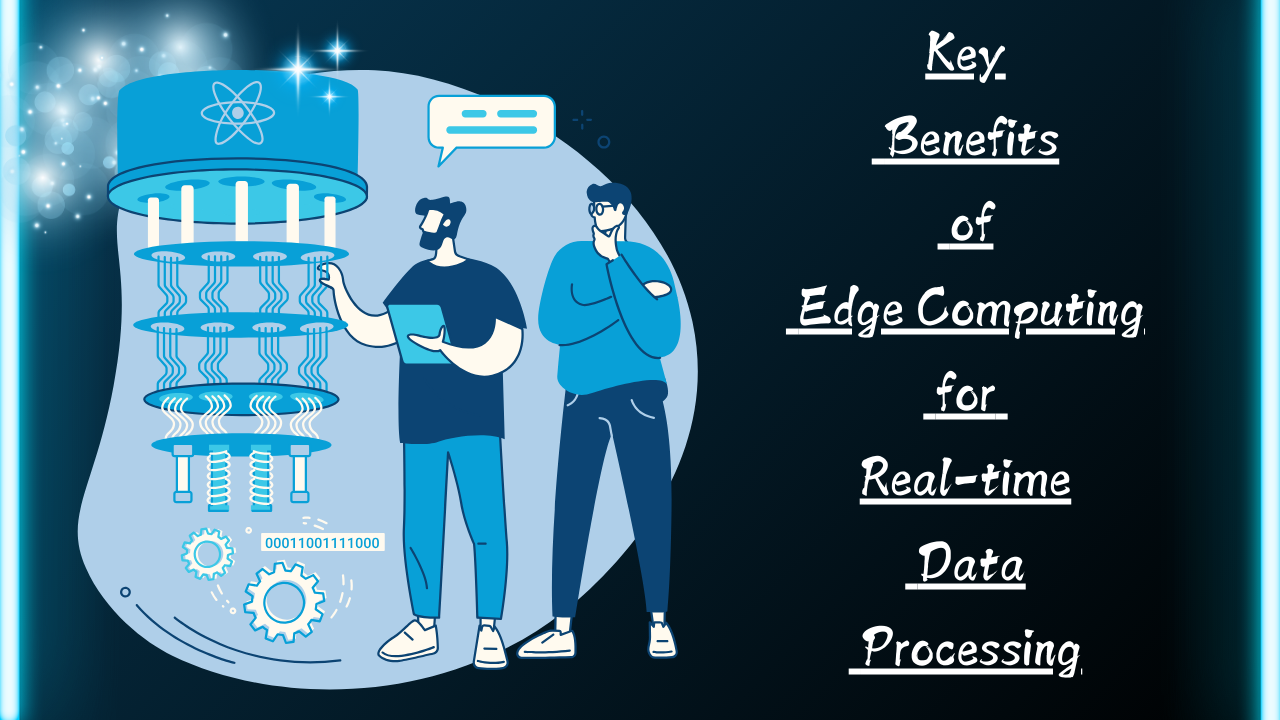
Reduced Latency
One of the most significant advantages of it is the reduction in latency. By processing data locally, edge devices eliminate the need to send large amounts of data to centralized cloud servers for processing. This is especially important for time-sensitive applications like autonomous driving and healthcare monitoring, where even a few milliseconds of delay can have severe consequences.
Enhanced Security and Privacy
It reduces the need for sensitive data to travel across networks. This limits exposure to potential cyber threats and minimizes the risk of data breaches. Edge devices can also enforce stricter security measures, enhancing overall data protection.
Improved Reliability
Edge computing allows systems to operate independently of a central server. Even in cases where connectivity to the cloud is lost, edge devices can continue to function and process data locally, ensuring continuous operation and improved reliability.
Cost Efficiency
Processing data at the edge reduces the amount of data that needs to be transferred to the cloud, cutting down on bandwidth usage and associated costs. Businesses can also save on cloud storage and processing fees by keeping more data local.
Edge Computing vs. Cloud Computing: What’s the Difference?
Cloud computing relies on large, centralized data centers that handle most of the processing tasks. While this model works well for many applications, it introduces latency and requires constant data transmission between devices and the cloud. In contrast, edge computing processes data closer to the source, reducing latency and improving efficiency, particularly in real-time applications.
Use Cases of Edge Computing in Real-time Data Processing
Manufacturing and Industrial IoT
In manufacturing, real-time monitoring of machinery and production lines is essential for optimizing efficiency and preventing equipment failure. Edge computing enables real-time analytics on production data, allowing machines to automatically adjust operations or alert operators to potential issues.
Autonomous Vehicles
Autonomous vehicles rely heavily on data from sensors to navigate and make decisions. Edge computing allows these vehicles to process data in real time, ensuring quick responses to changes in the environment, such as pedestrians crossing the road or other vehicles changing lanes.
Healthcare and Telemedicine
Edge computing is revolutionizing healthcare by enabling real-time monitoring of patients through wearable devices and IoT sensors. This allows for immediate feedback on patient conditions, especially in critical situations, reducing the need for data to be sent to distant servers for analysis.
Smart Cities and Infrastructure
Smart cities rely on real-time data to manage traffic, monitor air quality, and optimize energy usage. Edge computing allows these systems to operate with minimal delays, ensuring that infrastructure responds to changing conditions immediately.
Retail and Customer Experience
edge computing can enhance customer experience by processing data from IoT devices in real-time, such as smart shelves, checkout systems, and personalized marketing displays.
The Role of AI and Machine Learning in Edge Computing
Artificial intelligence (AI) and machine learning (ML) are playing a significant role in enhancing it. By integrating AI models at the edge, devices can make intelligent decisions without needing to communicate with the cloud. For example, AI-powered edge cameras can analyze video feeds in real-time to detect security threats or monitor customer behavior in a retail environment.
Challenges in Implementing Edge Computing
Data Privacy and Security
While edge computing can enhance security by limiting the exposure of sensitive data, it also introduces new risks. Securing a large number of edge devices, often located in remote or unsecured environments, can be challenging.
Infrastructure and Scalability
Building the infrastructure required to support edge computing can be complex and costly. Scaling its system to manage thousands or millions of devices requires careful planning and robust management systems.
Power and Resource Constraints
Many edge devices have limited power and computational resources. Ensuring that these devices can perform complex data processing tasks efficiently without draining battery life is a challenge.
Future Trends
The future of edge computing will likely see greater integration with AI and ML, enabling even more advanced real-time decision-making capabilities. We can also expect to see it become more widespread in industries such as agriculture, energy, and entertainment as businesses seek to leverage real-time data processing to improve operations.
Edge Computing in Energy and Utilities
Another area where it is making significant strides is in the energy and utilities sector. With the rise of smart grids and renewable energy sources, managing the flow of electricity and optimizing energy usage has become more complex. It enables utilities to process data from smart meters, sensors, and other grid devices in real time, allowing for better load balancing, outage detection, and energy distribution. This real-time responsiveness is crucial for maintaining a stable energy supply, reducing costs, and integrating renewable energy sources like solar and wind, which can be unpredictable.
In addition, It can play a critical role in improving energy efficiency at both residential and industrial levels. Smart thermostats, lighting systems, and appliances can make autonomous adjustments based on real-time data processing at the edge, further enhancing energy savings.
Edge Computing in Agriculture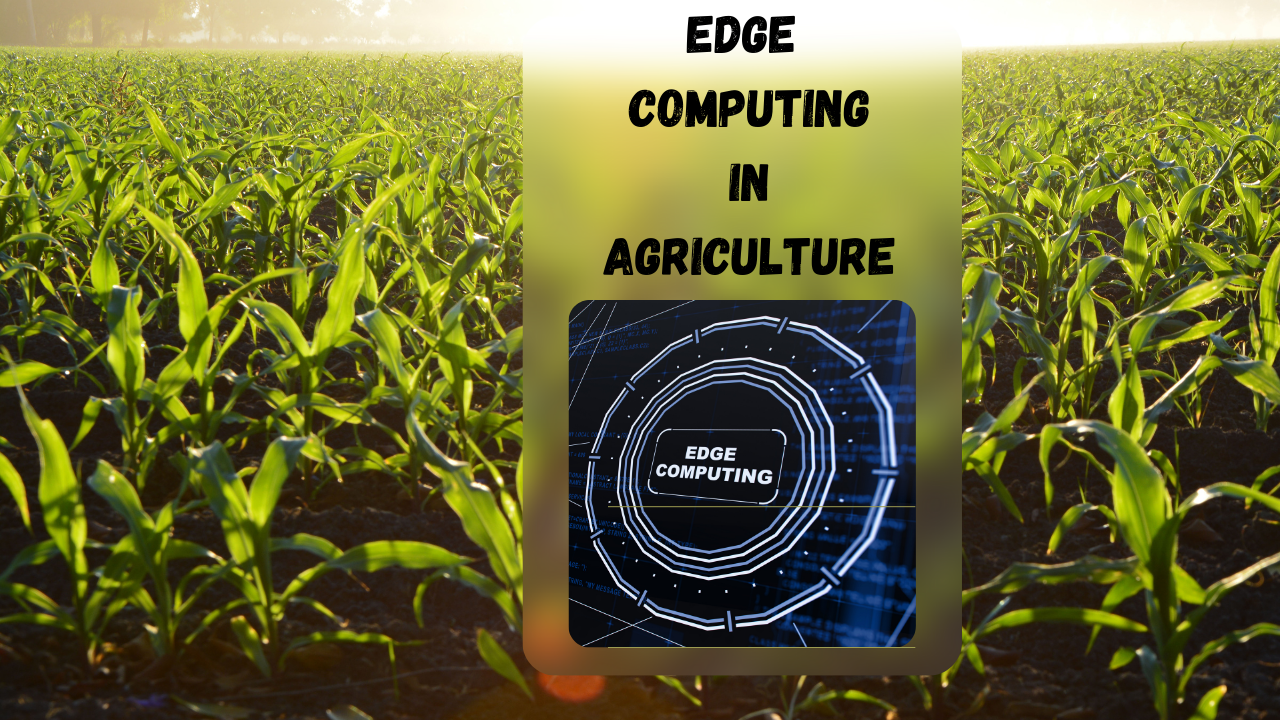
The agricultural sector is also leveraging edge computing to drive efficiency and sustainability. Precision agriculture, which relies on data from soil sensors, drones, and weather stations, can benefit immensely from real-time data processing. It allows farmers to get instant insights into soil conditions, moisture levels, and crop health, enabling them to make immediate decisions about irrigation, fertilization, and pest control.
By processing this data locally, edge computing reduces the need for constant connectivity in rural areas, where internet infrastructure may be lacking. This ensures that farmers can still optimize their operations using real-time data, even in remote locations.
Conclusion:
As edge computing continues to evolve, its role in real-time data processing across industries will only grow. The technology offers immense potential for improving efficiency, reducing latency, and enabling faster, smarter decision-making. Whether it’s powering smart cities, optimizing industrial operations, or enhancing healthcare delivery, edge computing is set to become an indispensable part of the modern technological landscape. As 5G networks and AI further integrate with it, the possibilities for innovation and transformation in data processing will continue to expand.
FAQs
What is edge computing?
Edge computing refers to the practice of processing data at or near the source, rather than relying on centralized cloud servers.
How does edge computing benefit real-time data processing?
It reduces latency by processing data locally, which is crucial for time-sensitive applications such as autonomous vehicles and healthcare.
What are the key challenges in implementing edge computing?
Challenges include securing data on numerous edge devices, building scalable infrastructure, and managing resource constraints.
How does edge computing differ from cloud computing?
Cloud computing relies on centralized data centers. Edge computing processes data closer to the source, reducing the need for constant communication with the cloud.
What are some examples of edge computing in use today?
It is famous in autonomous vehicles, smart cities, healthcare monitoring, and industrial IoT applications.